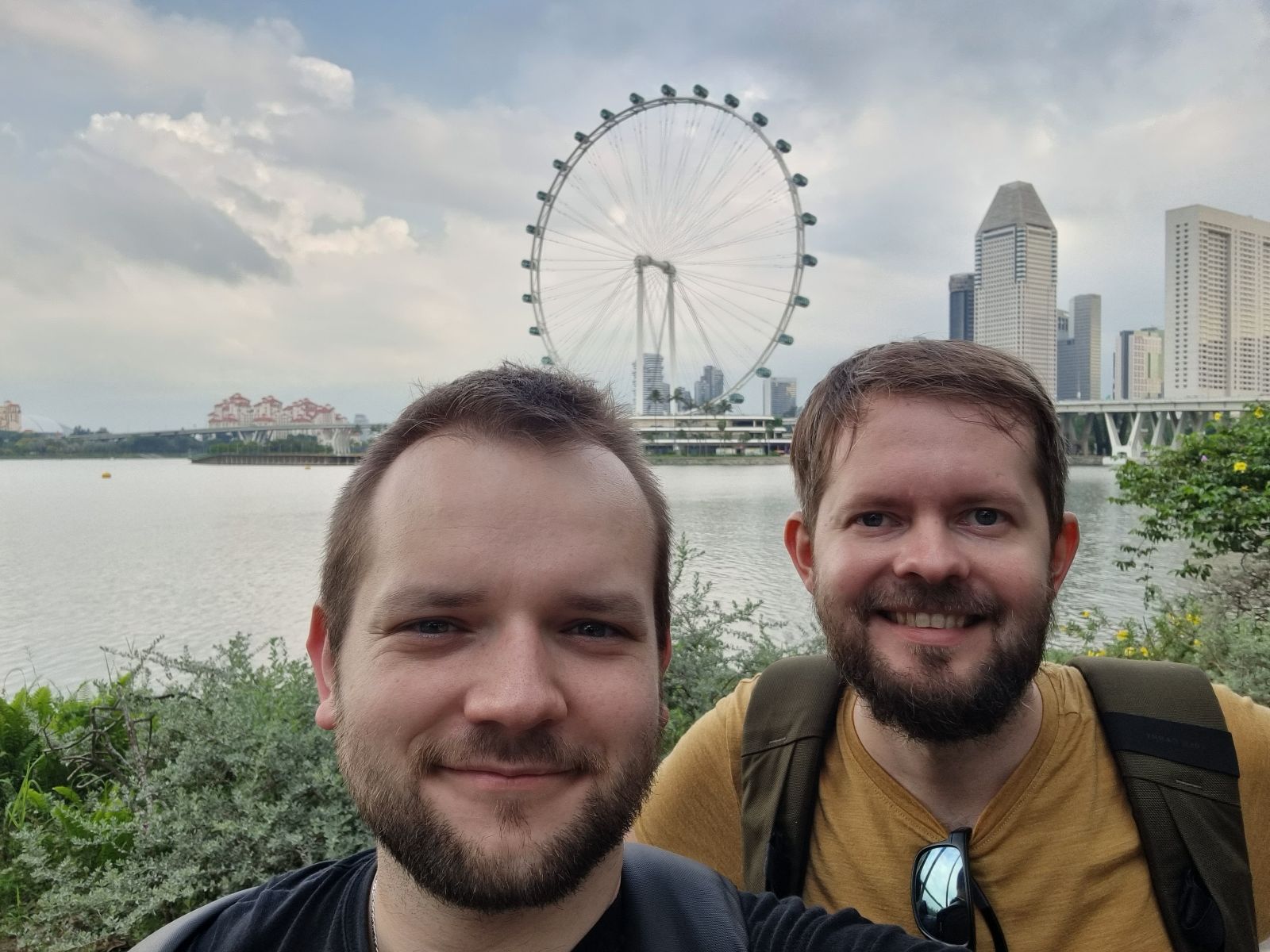
Two of the VIGILANT project consortium members, the Kempelen Institute of Intelligent Technologies (KInIT) and the University of Sheffield presented three papers at the EMNLP conference – one of the most prestigious events within the domain of Natural Language Processing (NLP).
This year's EMNLP held in Singapore drew over 2000 NLP experts from around the world, providing a convenient platform for our partners to not only present their cutting-edge research but also to highlight the collaborative efforts under the VIGILANT project.
"The EMNLP conference was a great opportunity to see the current trends in NLP first hand, see interest in our work on machine-generated text detection, get feedback on it and draw inspiration for our continued work on the VIGILANT project." Róbert Móro, Senior Researcher at KInIT
One of the papers presented by our partners, developed in direct collaboration with the VIGILANT project, addressed a burgeoning challenge in the NLP community: detecting machine-generated text. This research is particularly pertinent in an era where distinguishing between human and artificial text is becoming increasingly crucial. In light of this, the paper introduces a benchmarking dataset named 'MULTITuDE', designed specifically to tackle this issue.
The 'MULTITuDE' dataset is a significant advancement in the field, as it facilitates the comparison of various existing methods for detecting machine-generated text. It serves as a valuable tool for both researchers and practitioners, aiding in the development of more sophisticated and accurate detection algorithms. The dataset stands out for its comprehensive coverage, encompassing a range of languages, which is crucial for developing universally applicable NLP tools.
Moreover, 'MULTITuDE' supports the progression of research in understanding and identifying machine-generated content. By providing a rich and diverse set of data points, it enables a deeper analysis of the effectiveness of different detection methods. This contribution is pivotal in enhancing the reliability and robustness of NLP applications, ensuring they remain effective in the face of rapidly evolving machine-generated text technologies.
For a deeper dive into the paper's findings and methodology, read the full version here.